Banking and Finance
here's plenty of big data in every industry, especially banking and financial services. Except for dispensing cash from ATMs, there's nothing tangible-every customer interaction simply generates electronic records that must be retained due to regulatory requirements. Thanks to big data analytics, financial services firms are no longer simply storing data as required; they're actively using it in order to generate business insights and add value. They aren't waiting to conduct historical analyses, either. Most of the big data analytics that these businesses perform happen in real time to drive immediate decision-making. Here are five of the most common use cases where banks and financial services firms are finding value in big data analytics.Sample Architecture
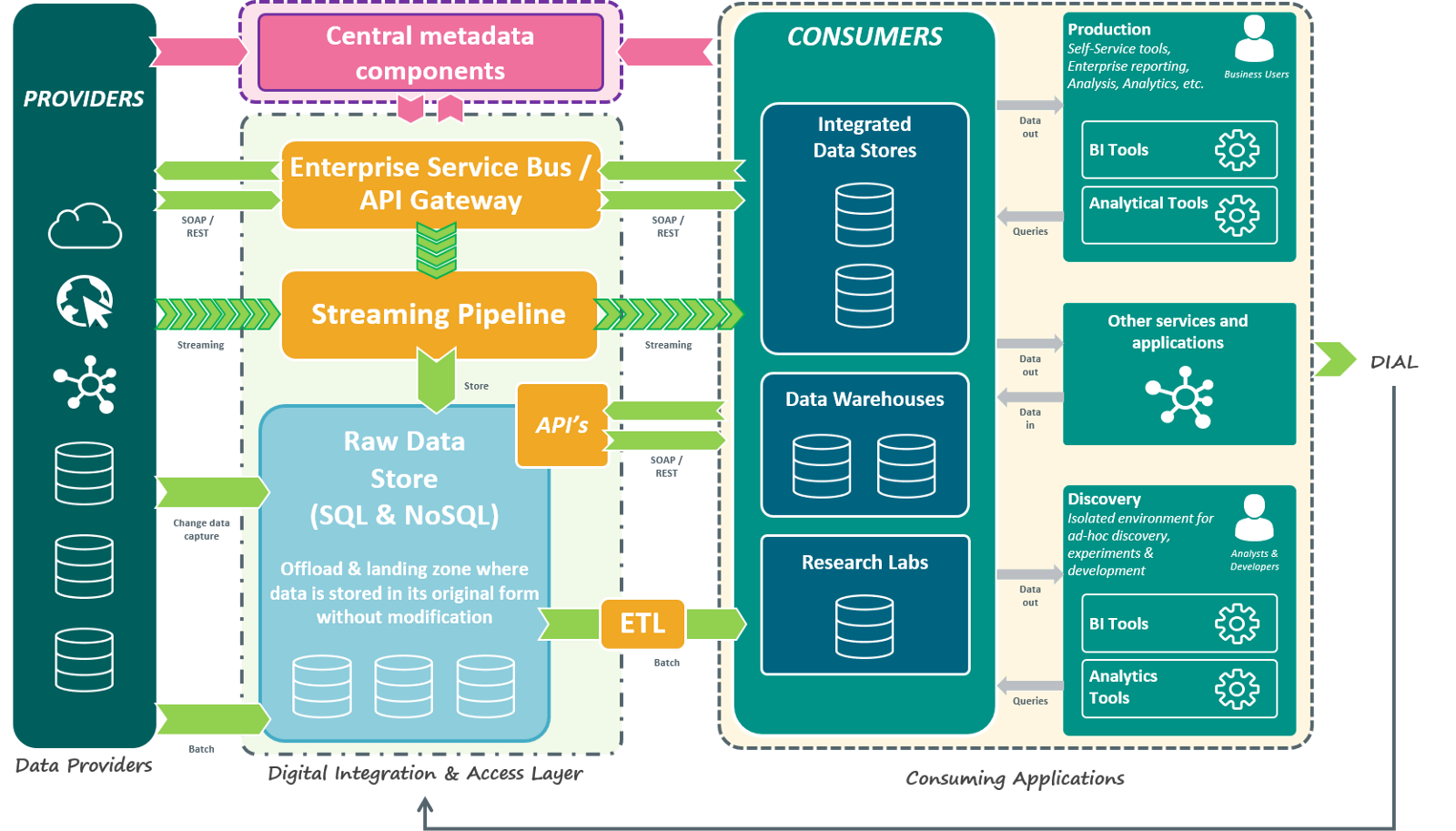